Emotion analysis, also known as sentiment analysis, is a powerful AI-driven tool that enables businesses to gauge customer sentiment by analyzing their communications and interactions. Using natural language processing and machine learning techniques, this technology dives into text and audio, such as social media posts, reviews, or surveys, to identify the emotional tone behind them. By distinguishing positive, negative, or neutral sentiments present in customer communications, companies can make more informed decisions to enhance their product offerings, services, and overall customer experience.
One notable advantage of emotion analysis is its ability to make customer touchpoints provide valuable insights in real time. Traditional methods like surveys and feedback forms often need to catch up when capturing a comprehensive picture of customer emotions at any given moment. This AI-powered approach identifies customer sentiments faster and more efficiently, giving companies a better understanding of their audience’s emotions and promptly addressing any grievances or concerns.
Companies across various industries embrace emotion aspect-based sentiment analysis to improve their customer experience. For instance, restaurants use it to fine-tune their menus and services based on online reviews, while e-commerce platforms utilize AI-generated recommendations that consider customer sentiment.
Even airlines apply this technology to analyze social media data and enhance in-flight experiences accordingly. Through sentiment analysis, businesses foster a climate of empathetic understanding, paving the way for personalized and satisfactory customer experiences.
- Foundations of Emotion Analysis
- Implementing AI in Customer Sentiment Analysis
- Practical Applications of Emotion Analysis
- Ethical and Cultural Considerations in Emotion Analysis
Foundations of Emotion Analysis
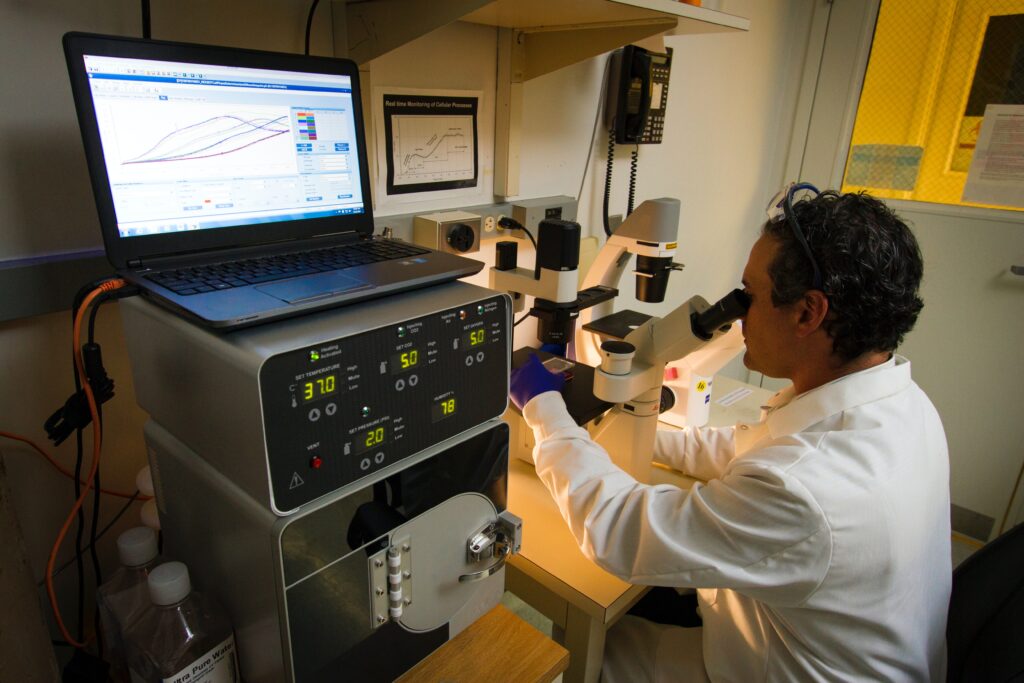
Understanding Emotions and Sentiment
Human emotions are crucial in expressing our experiences and reactions to different stimuli. An analysis of sentiment, or opinion mining, is a method to detect feelings, opinions, and attitudes in text-based data using artificial intelligence (AI) and machine learning (ML). In sentiment analysis, the primary goal is polarity detection, determining whether a message expresses positive, negative, or neutral sentiment.
AI uses neural networks, natural language processing (NLP), and deep learning to identify emotions and opinions in text, audio, and visual data. Sentiment analysis models assist companies in understanding customer sentiment and uncovering valuable information from unstructured sources, such as social media interactions, customer reviews, and support tickets, by identifying emotions.
Emotion AI and Sentiment Analysis Tools
Emotion AI is a subfield of affective computing that strives to recognize, understand, and respond to human emotions. With the help of ML and NLP techniques, emotion AI empowers businesses to analyze emotional cues, facial expressions, and tonality in their customer interactions, facilitating empathetic and effective communication.
Sentiment analysis tools come in various forms and offer different levels of sophistication. Some key features that most sentiment analysis tools possess are:
Text analysis: The ability to process and analyze textual data, such as written reviews or social media posts, by identifying keywords, phrases, and patterns that indicate emotions and opinions.
Voice analysis: The capacity to evaluate spoken language by analyzing factors such as pitch, tone, and tempo to discern emotional cues in customer phone calls, voice messages, and video conversations.
Image analysis: The capability to interpret visual elements, including facial expressions and gestures, to identify emotions in photos, videos, and other visual content.
Using emotion AI and sentiment analysis tools to monitor, analyze, and respond to customers’ emotions in existing business processes, workflows, and customer management systems can improve customer satisfaction, customer experience, and brand perception by improving customer satisfaction, user experience, and brand perception.
Implementing AI in Customer Sentiment Analysis
Data Collection and Processing
To effectively analyze customer sentiment using AI, the first step involves collecting and processing various customer data. This may include:
Textual data: Online reviews, social media posts, customer support interactions, and survey responses.
Audio data: Call center recordings, voice responses, etc.
Employing natural language processing (NLP) techniques, the raw data is transformed into meaningful metrics that help evaluate key performance indicators and customer satisfaction. Common processes involve feature extraction and converting the data into a format that AI algorithms can utilize.
Building Accurate Sentiment Analysis Models
Developing AI models for sentiment analysis requires a sizable dataset for training and testing purposes. This dataset should encompass the following:
Positive sentiment: Indicative of satisfaction and appreciation
Negative sentiment: Signifying dissatisfaction or disappointment
Neutral sentiment: Denoting the absence of strong emotions or simply providing information
Incorporating big data and advanced AI techniques, such as deep learning, helps create reliable models capable of handling complex language patterns and diverse expressions.
During the model-building process, emphasis should be placed on:
Ensuring the model has been trained on a representative dataset covering various industries, demographics, and language styles.
Continuously refining and updating the model to account for evolving communication trends and sentiment indicators.
Real-Time Analysis and Actionable Insights
One of the key advantages of AI-driven sentiment analysis is the ability to mine customer data platforms to gain real-time insights into customer emotions. By processing large volumes of customer feedback swiftly and accurately, businesses can:
Proactively address customer concerns and improve the overall customer experience.
Make data-driven decisions based on customer feedback to enhance products/services and develop effective marketing strategies.
Moreover, real-time sentiment analysis empowers customer support teams to:
Identify potentially dissatisfied customers and respond proactively to resolve issues, thereby increasing customer satisfaction.
Direct customers to relevant support channels, optimizing the use of resources and providing targeted assistance.
To sum up, effectively implementing AI in customer sentiment analysis involves collecting and processing relevant data, creating accurate models to gauge emotions, and transforming these insights into actionable strategies. This ultimately results in predictive analytics and a better understanding of customer needs, fostering an enhanced customer experience.
Practical Applications of Emotion Analysis
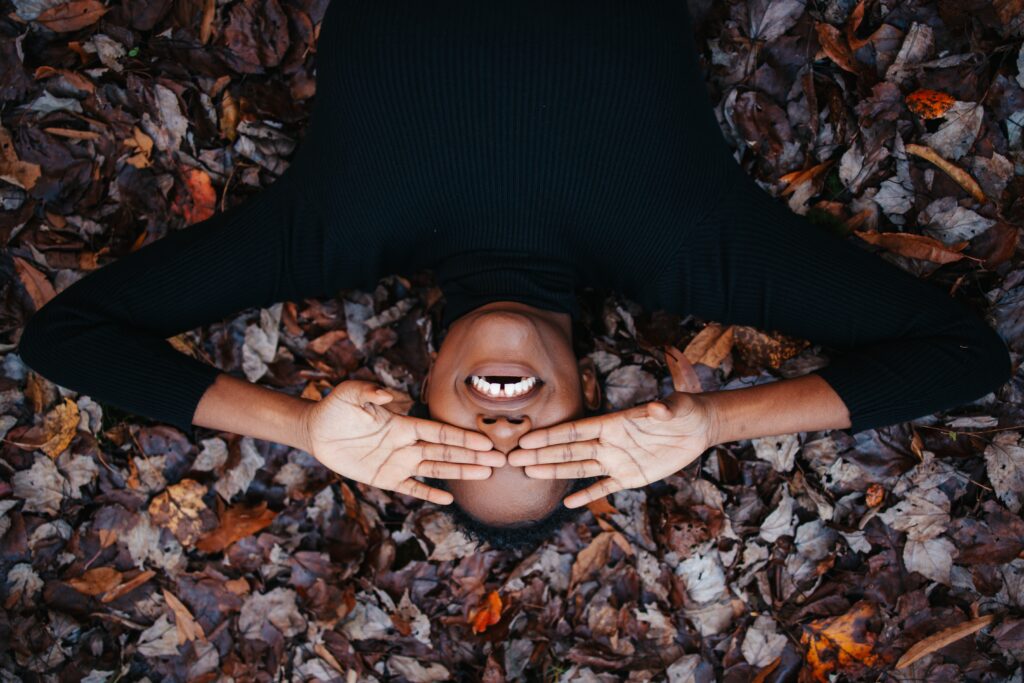
Marketing and Customer Experience Enhancement
Emotion analysis using AI can significantly improve marketing efforts and customer experience management by identifying customer behavior and sentiment patterns. This can be done by monitoring various customer lifetime channels, like social media, product reviews, and customer support interactions. Insights from emotion analysis can be used to tailor marketing campaigns, resulting in more targeted and effective communication with customers.
Some ways in which emotion analysis can be applied to marketing and customer experiences include:
Identifying customer sentiments toward specific products or services
Analyzing consumer behavior during different stages of the purchase process
Personalizing advertisements and promotions based on the emotional state of customers
This enhanced understanding of customer emotions allows businesses to create more engaging and memorable experiences to engage customers, ultimately contributing to increased loyalty and customer satisfaction.
Business Intelligence and Competitive Advantage
Emotion analysis can provide valuable insights into current market trends and customer preferences, allowing businesses to stay ahead of their competitors. By keeping track of customer sentiments and their impact on brand reputation, companies can identify areas of the customer journey that need improvement and allocate resources more effectively.
Incorporating emotion analysis into business intelligence efforts can lead to:
Improved Net Promoter Score (NPS)
Better understanding of customer needs and preferences
Increased brand loyalty and customer retention
By leveraging these insights, businesses can make informed decisions that drive growth and create a competitive advantage in the market.
Service Recovery and Customer Relations
Emotion analysis can also play a crucial role in identifying customer dissatisfaction early on and addressing it promptly. By monitoring customer engagement through various communication channels such as social media, reviews, and customer support records, businesses can detect negative sentiments and respond proactively to resolve issues.
Effective use of emotion analysis for customer service agents, recovery, and customer relations may include:
Recognizing patterns of dissatisfaction or complaints
Identifying the root causes of negative customer experiences
Facilitating timely and personalized responses to unhappy customers
Incorporating emotion analysis into customer relations efforts can help businesses mitigate negative experiences, foster better relationships with their customers, and, ultimately, maintain a strong brand reputation.
Ethical and Cultural Considerations in Emotion Analysis
Privacy and Ethical Use of AI
Emotion analysis using AI raises several privacy and ethical concerns. Companies like Affectiva and Cogito specialize in extracting customer sentiment through their communications and interactions. However, the question of how to handle sensitive emotional data responsibly arises.
To understand customer expectations, companies must ensure the ethical use of AI-based emotion detection and recognition technologies. There are three key areas of ethical concern, as found in a review of the literature: First, the risk of biased and unfair outcomes due to the faulty bases and problematic premises of Emotion Recognition Technologies (ERT); second, the sensitivity of emotion data used by ERT; and third, the risk of harm that arises from the technologies in consequential settings, such as employment and education.
Moreover, the ethical considerations extend to factors such as:
Ensuring data privacy by anonymizing collected data
Obtaining informed consent from customers
Using secure storage and data transmission methods
Adhering to data protection regulations
Accounting for Cultural Differences
Another critical aspect of emotion analysis is accounting for cultural differences. Human emotions are complex and can be influenced by an individual’s culture and other social factors. This means that the application of emotion recognition technologies should consider the various nuances and variations in cultures.
Emotion analysis should be grounded in the understanding that cultural differences can impact the interpretation and perception of emotions. In this context, AI algorithms must be:
Designed to recognize and handle emotions across diverse cultural contexts
Continuously updated to reflect changes in cultural norms and customer expectations
By aligning ERTs with cultural sensitivities and respecting both the customer and privacy concerns associated with emotion data, companies can provide better customer experiences. Ultimately, striking a balance between utilizing AI to analyze customer sentiment and respecting individual privacy and cultural differences remains a challenge that requires continuous evaluation and refinement.
Conclusively, Platforce is the best CRM software for lead generation, order management, sales automation, and task management. Unlike other software, Platforce gives your company all it needs to optimize customer relations and boost customer experience. Try out the Platforce CRM system with a demo or tour now. You can also stay updated on our Youtube and LinkedIn channels.